Abstract
Thermography is especially valuable for the military and other users of surveillance cameras. Some recent methods based on Neural Radiance Fields (NeRF) are proposed to reconstruct the thermal scenes in 3D from a set of thermal and RGB images. However, unlike NeRF, 3D Gaussian splatting (3DGS) prevails due to its rapid training and real-time rendering. In this work, we propose ThermalGaussian, the first thermal 3DGS approach capable of rendering high-quality images in RGB and thermal modalities. We first calibrate the RGB camera and the thermal camera to ensure that both modalities are accurately aligned. Subsequently, we use the registered images to learn the multimodal 3D Gaussians. To prevent the overfitting of any single modality, we introduce several multimodal regularization constraints. We also develop smoothing constraints tailored to the physical characteristics of the thermal modality. Besides, we contribute a real-world dataset named RGBT-Scenes, captured by a hand-hold thermal-infrared camera, facilitating future research on thermal scene reconstruction. We conduct comprehensive experiments to show that ThermalGaussian achieves photorealistic rendering of thermal images and improves the rendering quality of RGB images. With the proposed multimodal regularization constraints, we also reduced the model’s storage cost by 90%. Our project page is at https://thermalgaussian.github.io/.
RGBT-Scenes Dataset
The following 10 scenes were selected for both qualitative and quantitative analysis in our paper.
Scene | RGB | Thermal | MSX | Views | Temp. Range |
---|---|---|---|---|---|
Dimsum | ![]() |
![]() |
![]() |
134(train) 20(test) | 23.1°C - 60.0°C |
Daily Stuff | ![]() |
![]() |
![]() |
68(train) 10(test) | 17.5°C - 56.3°C |
Electric Bicycle | ![]() |
![]() |
![]() |
42(train) 6(test) | 14.5°C - 18.5°C |
Roadblock | ![]() |
![]() |
![]() |
62(train) 9(test) | 22.0°C - 27.0°C |
Truck | ![]() |
![]() |
![]() |
64(train) 9(test) | 30.6°C - 249.0°C |
Rotary Kiln | ![]() |
![]() |
![]() |
92(train) 14(test) | 5.0°C - 60.4°C |
Building | ![]() |
![]() |
![]() |
238(train) 35(test) | 15.0°C - 24.0°C |
Iron ingot | ![]() |
![]() |
![]() |
53(train) 8(test) | 38.0°C - 350.0°C |
Parterre | ![]() |
![]() |
![]() |
57(train) 9(test) | 19.5°C - 27.5°C |
Landscape | ![]() |
![]() |
![]() |
90(train) 13(test) | 16.0°C - 23.0°C |
RGBT-Scenes-extend-Dataset
The following are the four scenes extended by RGBT-Scenes.
Scene | RGB | Thermal | MSX | Views | Temp. Range |
---|---|---|---|---|---|
Glass Cup | ![]() |
![]() |
![]() |
123(train) 18(test) | 17.0°C- 36.6°C |
Transmission Tower | ![]() |
![]() |
![]() |
154(train) 23(test) | -26.4°C- 23.7°C |
Dark Scene | ![]() |
![]() |
![]() |
75(train) 11(test) | 17.5°C- 21.6°C |
Plant Equipment | ![]() |
![]() |
![]() |
192(train) 28(test) | 27.8°C- 54.9°C |
Dynamic scene demo
We also provide four dynamic scenes demos for our upcoming work to progress.
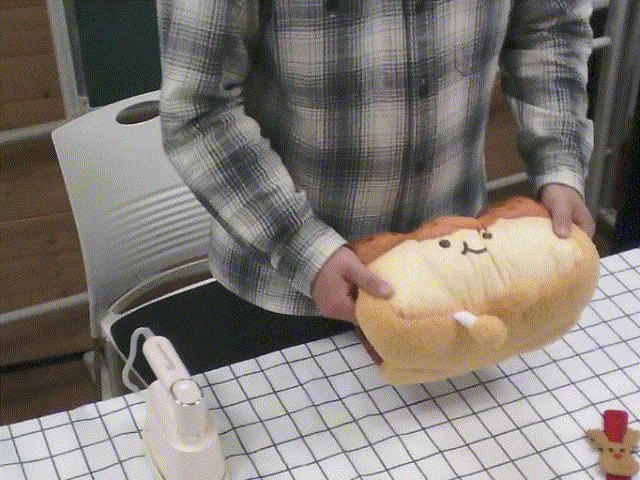
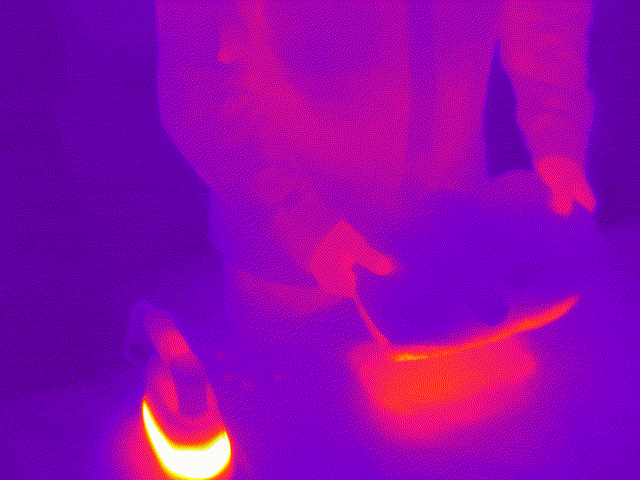
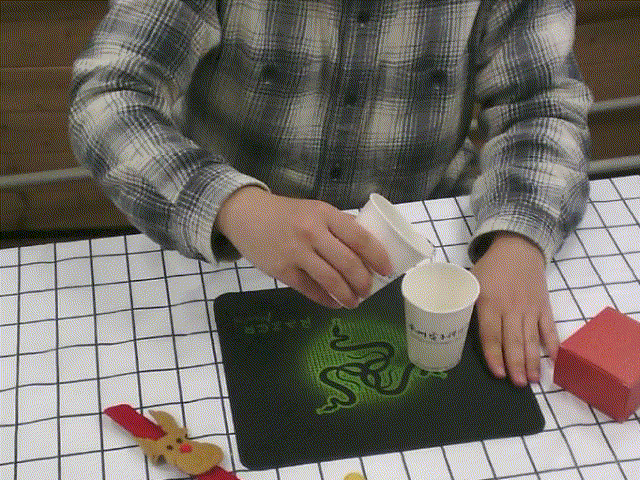
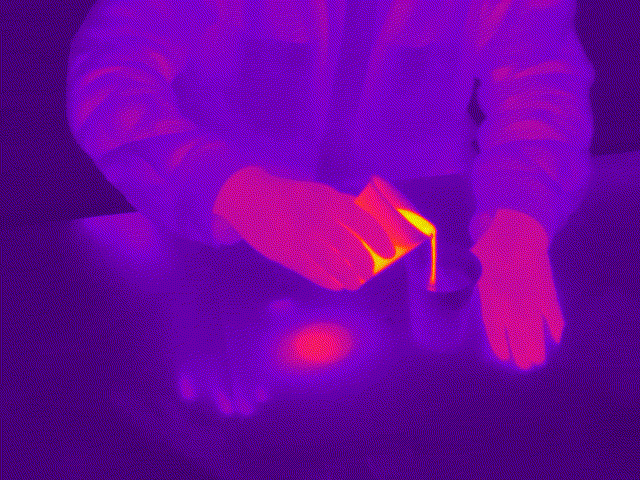
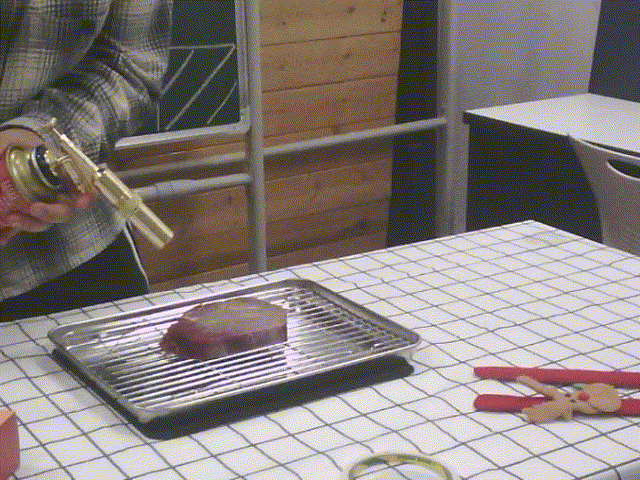
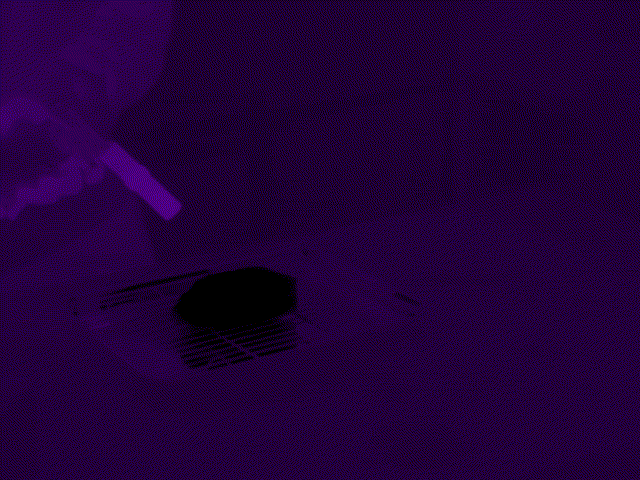
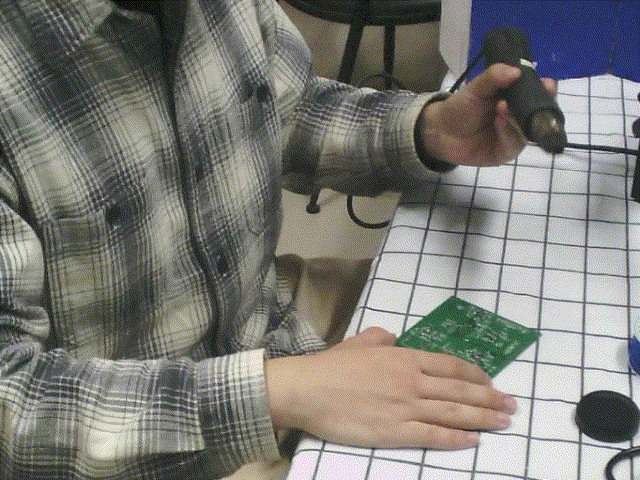
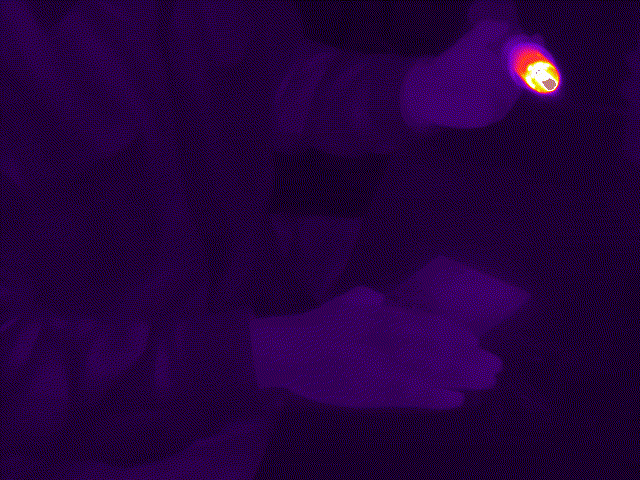
Comparisons
We present qualitative thermal image comparisons between our method, previous approaches (3DGS and ThermoNerf) , and the corresponding ground truth images from test views. We also show the training results of the MSX images, which are easier to apply.
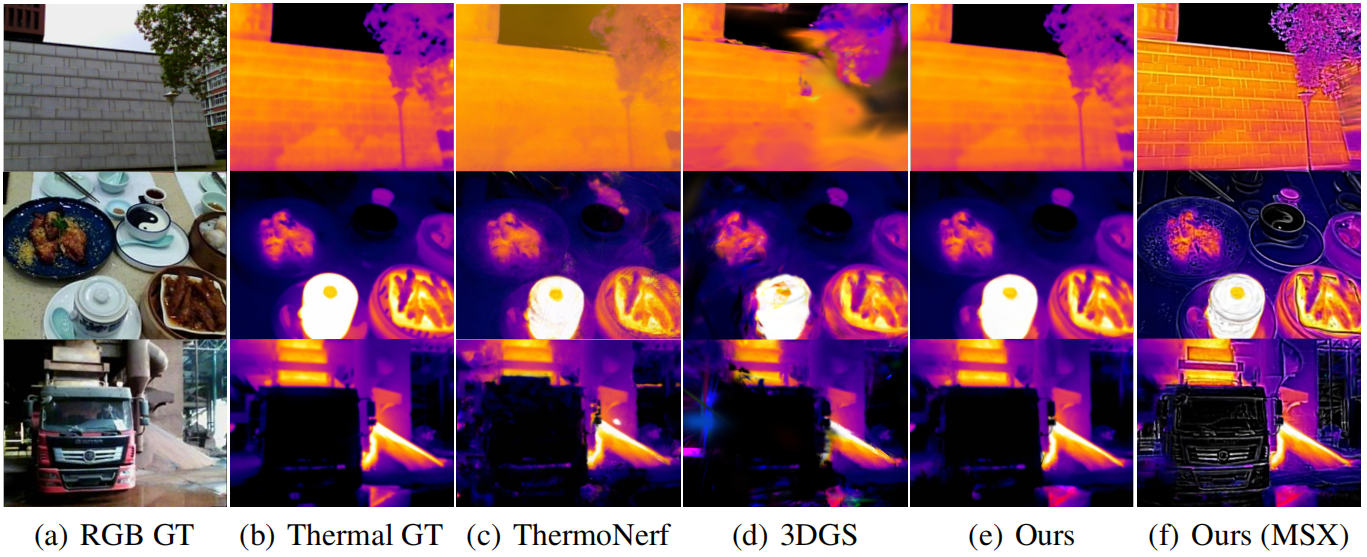
We present qualitative RGB image comparisons between our method and 3DGS from test views.
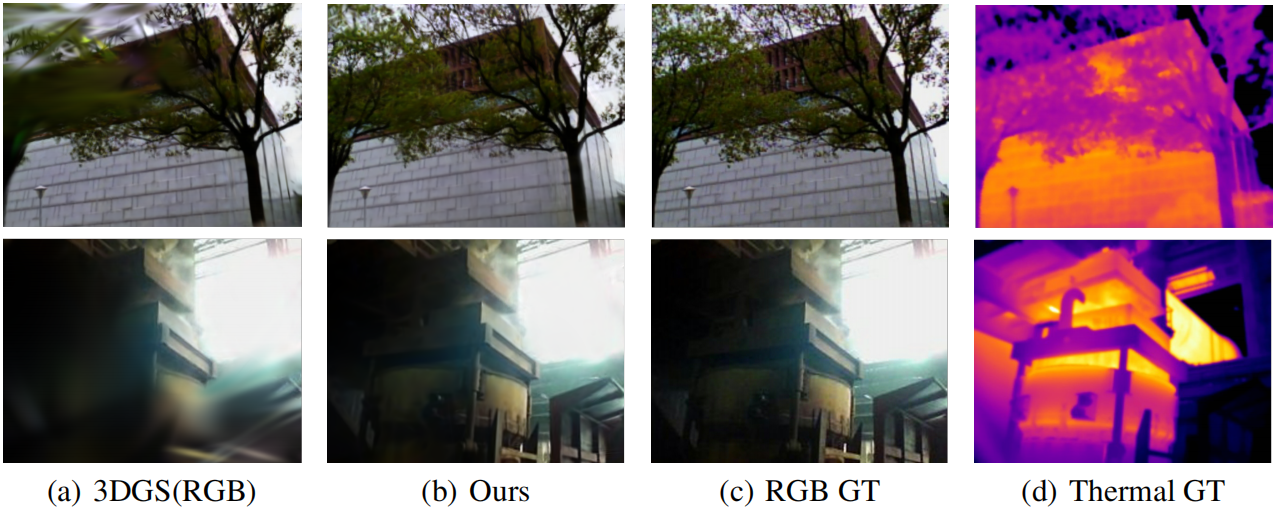
To verify the effectiveness of our multimodal regularization term, we compare our multimodal regularization γ with manual adjustment of the thermal constraint coefficient in a truck scene. The comparison shows that our multimodal regularization approach reduces the storage space of RGB and thermal modalities while maintaining high image quality. At the same time, we visually present the Gaussian distributions of the original 3DGS method and our method with multimodal regularization.
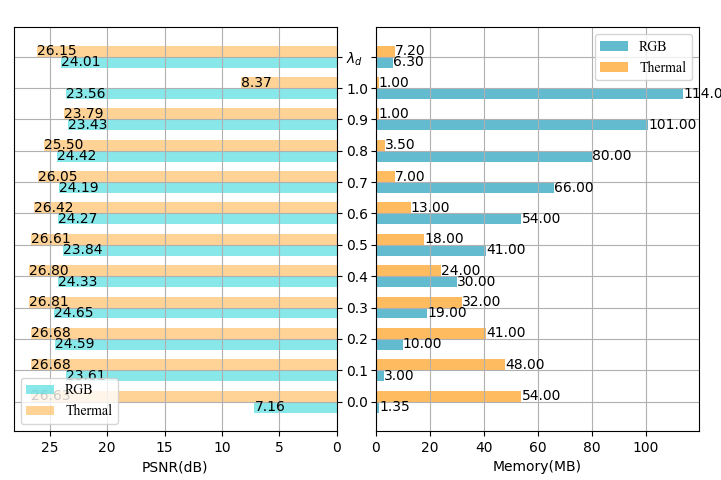
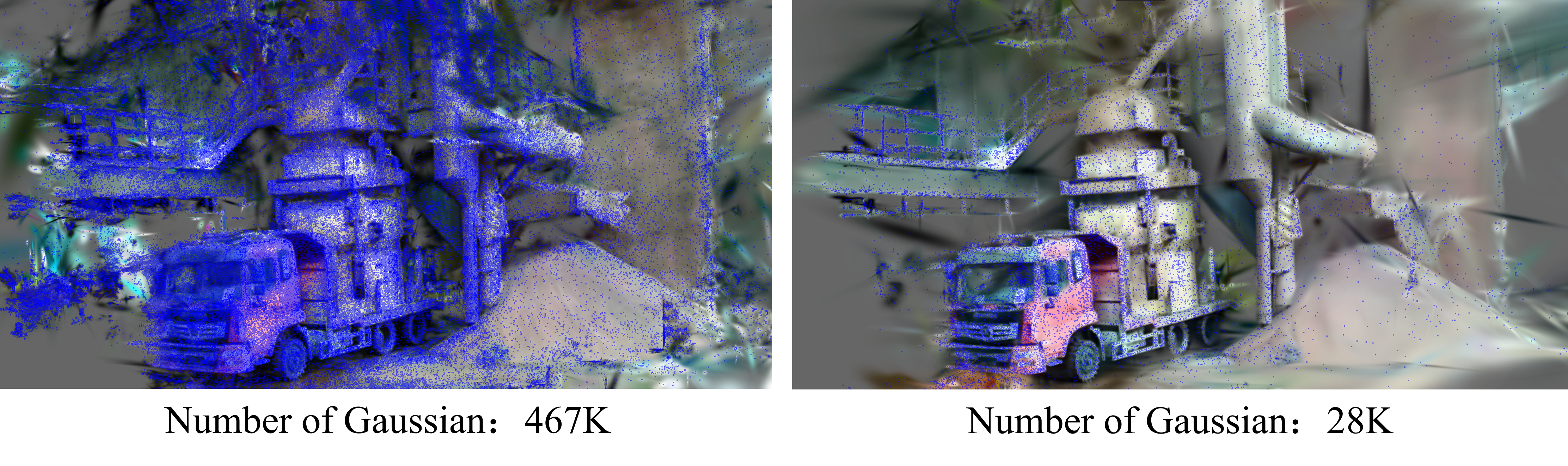
Citation
@article{lu2024thermalgaussian, title = {ThermalGaussian: Thermal 3D Gaussian Splatting}, author = {Lu, Rongfeng and Chen, Hangyu and Zhu, Zunjie and Qin, Yuhang and Lu, Ming and Zhang, Le and Yan, Chenggang and Xue, Anke}, journal = {arXiv preprint arXiv:2409.07200}, year = {2024}, }